Three Good Books to Teach You Data Skepticism
Three Good Books to Teach You Data Skepticismon Apr 28, 2021
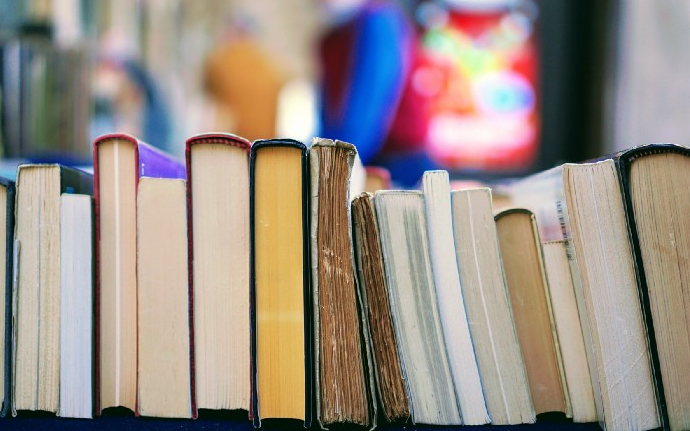
Most data science and machine learning education curriculums I’ve seen follow a similar pattern: either extract as many insights as possible from your data or maximize your accuracy on the validation set.
This approach rests on some key questions: 1) Are the data trustworthy in the first place? 2) Are the findings you collect meaningful? 3) What are the consequences if you come to the wrong conclusions? The books listed here will help you zoom out a little and look at data wrangling in context.
1: How Not to Be Wrong: The Power of Mathematical Thinking by Jordan Ellenberg
Despite the book’s title, author Jordan Ellenberg doesn’t seek to persuade the math-averse to embrace mathematical thinking. Rather, his goal is to encourage the mathematically inclined to not accidentally misuse their knowledge.
The book opens with an episode from World War II. A statistician was tasked with deciding where to put armor on fighter planes. The US Air Force was collecting data regarding where its returning planes had bullet holes. Intuitively, the armor was to go over where aircraft were getting hit most often. However, the statistician assigned to this problem flipped it on its head: the data were only being gathered on planes that survived. Tellingly, the engines did not have many bullet holes because getting shot in the engine meant a downed plane. With this opening anecdote, the book launches into consequential real-world cases in which data — and even the math applied to it — don’t necessarily speak for themselves. A recurring theme throughout the book is that mathematics is simply a manipulation of numbers according to rules that are sometimes arbitrary and that it is rarely a source of ultimate truth. But, properly employed, math can steer us away from demonstrably wrong decisions.
Ellenberg goes on to document real cases of fallaciously applied mathematics. Examples include a statistically sound experiment in which a dead fish can predict whether a photo of a face shows a happy or sad person and why three-way elections usually don’t accomplish what you expect them to.
Data scientists can benefit from Ellenberg’s extended discussions about the dangers of too little data and too much data. Too little data increases the likelihood of being fooled by a random outcome, while too much of it increases the chances of finding spurious correlations. The book’s most significant takeaway is how badly our numerical intuition can lead us astray and how to recognize situations in which that can happen.
2: Calling Bullsh*t: The Art of Skepticism in a Data-Driven World by Carl T. Bergstrom and Jevin D. West
This book identifies itself as the 21st-century version of a much earlier work (How to Lie with Statistics). Having read both, I can vouch for this comparison.
When someone qualifies a claim with “According to the data…” or “A study has shown…” they may be sincere, but such a statement can often be window dressing for bad data science. The danger is that data are often less conclusive than people claim. Because “data-driven” can sound so superficially persuasive, the term is now overused to the detriment of public discourse.
A frequent refrain throughout the book is: “If something is too good or too bad to be true, it probably is.”
Most true correlations in data just aren’t that exciting. However, journalists and public relations departments have adverse incentives to over-dramatize findings to draw clicks or gain notoriety. This book does an admirable job exposing the manners in which “findings” can be exaggerated out of context and details the structural motives for why these overdramatizations (or “bullsh*t,” as the book calls them in the aggregate) occur regularly and frequently.
Despite their bellicose title, Bergstrom and West avoid vindictive condemnations, display plenty of humility, and encourage their readers to do the same. Indeed, their final chapter is an appeal to not be a know-it-all who disproves people’s statements simply for the sake of doing so.
3: The 9 Pitfalls of Data Science by Gary Smith and Jay Cordes
I felt this book was geared toward product managers and executives but have seen actual data scientists (myself included) make many of the mistakes listed here. Whereas the previous book (Calling Bullsh*t) discusses how pundits and other public figures use data to fool audiences, this one focuses on how individuals and organizations use data science to fool themselves. The allure of elaborate algorithms, powerful computers, and massive data sets compels us to put aside common sense with surprising frequency. These temptations make us forget the limitations and snares that render statistics and machine learning fallible.
The book is full of elucidatory anecdotes, both public and from the authors’ experiences. If you come from a business background and are dealing with data science and machine learning for the first time, or are a data scientist and don’t immediately recognize the traps listed in the table of contents — I recommend it.
Common Themes and Conclusion
Impressively, none of these books are targeted to a technical audience. How Not to Be Wrong assumes minimal mathematical background, Calling Bullsh*t is aimed at people who want to be informed participants in the democratic process, and The 9 Pitfalls of Data Science only assumes basic knowledge of the subject. For anyone with a technical background, these are eminently readable.
John Ioannidis’s paper “Why Most Published Research Findings Are False” is discussed at length in all three books. The heart of one of the biggest problems in research today, the replication crisis, revolves around attributing more power to statistics than it possesses, so it is naturally a pertinent point of dialogue.
The simultaneous ease of identifying correlation and the difficulty of proving causation is also rightfully addressed. No matter how many times we repeat the mantra “correlation is not causation,” it’s often too tempting to misuse data that provide artificial clarity to uncertain decisions.
Reversion to the mean is another common point, and for a good reason — it’s devilishly easy to be fooled by it.
I recommend all three books. Some counterintuitive concepts need to be studied several times before one grasps them. Data science and machine learning are powerful and valuable when employed correctly. However, a complete education in these endeavors must include the ability to quickly recognize when they are misused.
Source: towardsdatascience
Sorry! No comment found for this post.